Hyperfine Global develops software for life science data and network analysis
Network Analytics are being used in many fields. Hyperfine Global has developed software to: generate and extract novel populations based on interdependent variables with Multi-Layer Analysis; analyze professional literature from the perspective of additional fields with the Comprehension Normalization Method (CNM) for Language; and rapidly extract functionally related subnetworks from network data with CNM for Networks.
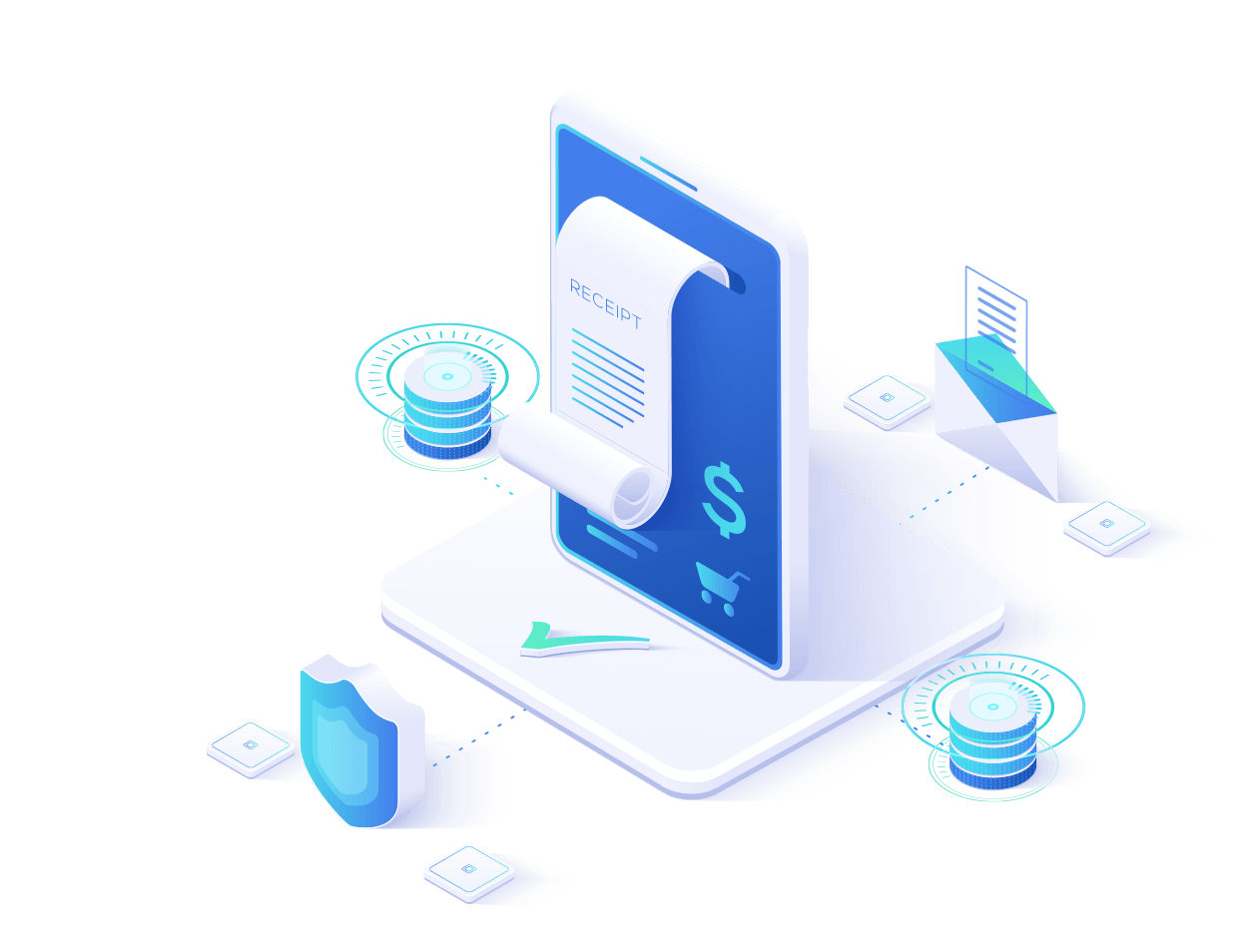
Software for Generating Novel Interdependent Factored Populations to Begin Prospective Research
Using both structured and unstructured data MLA enables the development of novel populations that account for the interdependent variables. MLA evaluates across layers, so instead of broadly applied criteria, this software enables the generation of novel populations by automating control-vs-variable analyses while weaving together different metrics, data, and criteria.
Software for Unstructured Semantic Data
Often technical literature is confined to a single discipline - resulting in siloed knowledge and a stifling of cross-disciplinary analysis. CNM for language evaluates and recombines pieces of literature from the perspective of different disciplines.
Software for Network Data
Networks help our customers study high dimensional data. Networks have consistent behaviors across fields. We are using it first for life science customers as they research parts of biology for the improvement of human health. The underlying technology can one day also be used in the fields of finance, defense, and advertising.
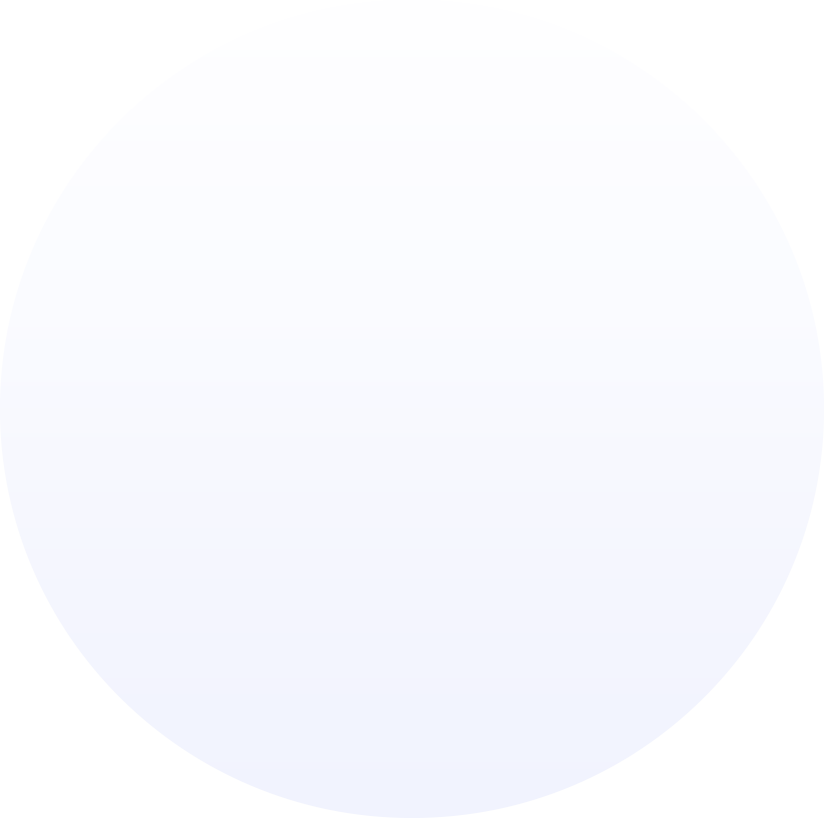
Hyperfine Global's Company History
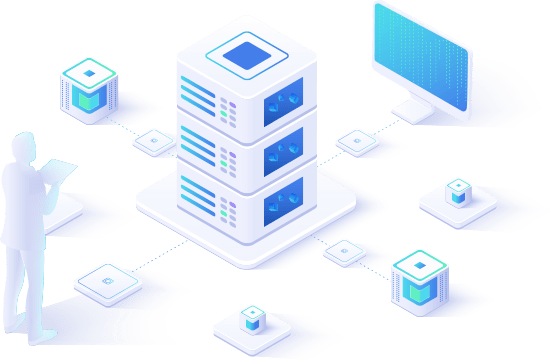
2014
Advisor Dr. Aaron Galaznik, then the Sr Director of Real World Data and Analytics at Pfizer – Joined our Scientific Advisory Board.
With him, we worked on our first technology Multi-Layer Analysis. We did preliminary work with Pfizer under Dr. Aaron Galaznik – Developed a crawler to gather Internet Data and built a tool demonstrating our capacity to work with unstructured data.

2015
Patent 1 (for Multi-Layer Analysis) Granted. The patent is a computer process designed to generate novel data populations by incorporating interdependent components across different data sets and conditions.
When applied to unstructured data, it is able to temporarily generate structure; auto-populating variables out of unstructured data to use as the next layer under evaluation.
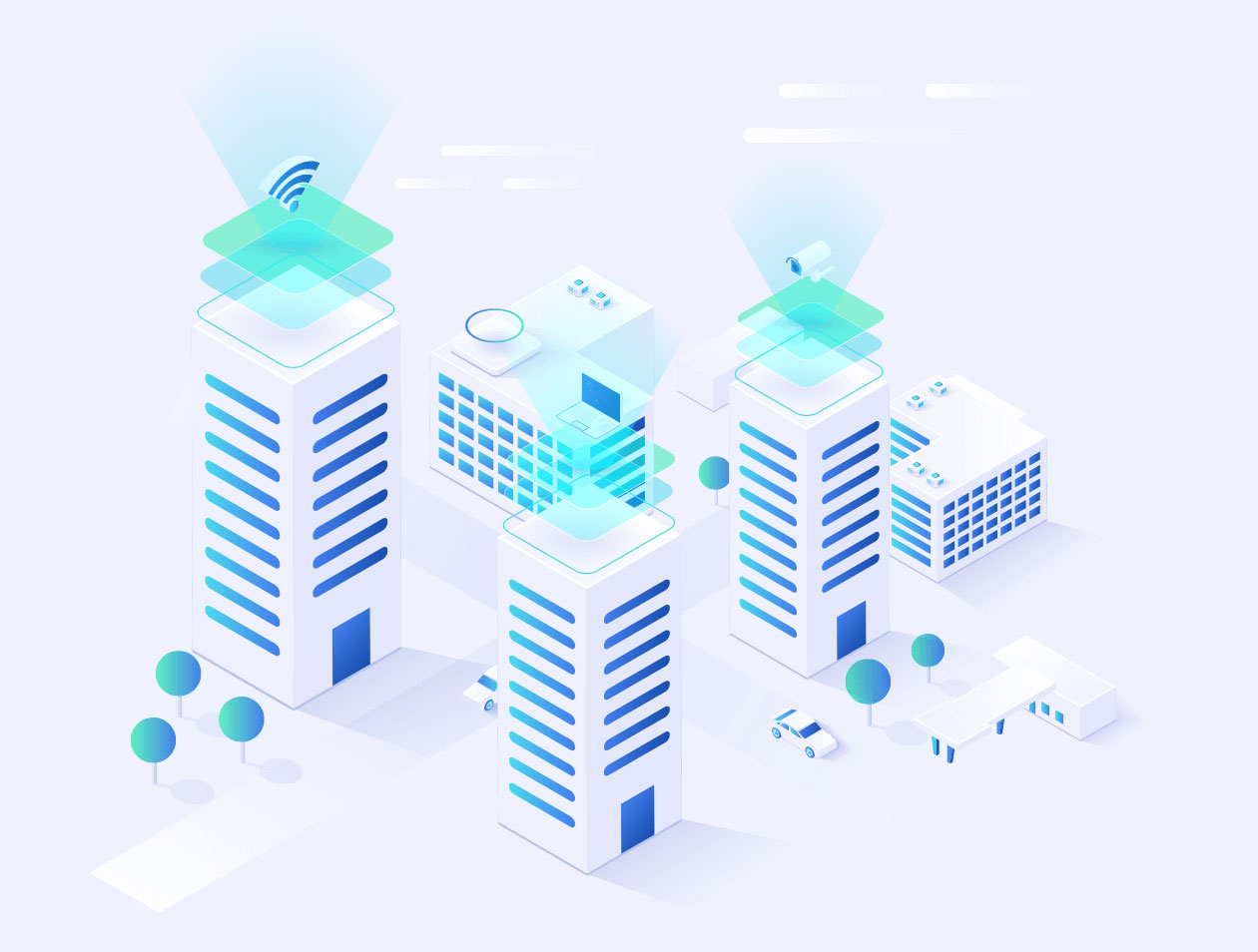
2015
Advisor Dr. Eric Schadt, then the Founding Director of Genomics and Multi-scale Biology at Mount Sinai, currently CEO of sema4 – joined our Scientific Advisory Board
Using data from his paper on multi-tissue coexpression networks, we performed with him our first validation of CNM. We were able to recreate the resulting subnetworks discovered in the paper, which likely originally took a matter of months, in a matter of minutes.
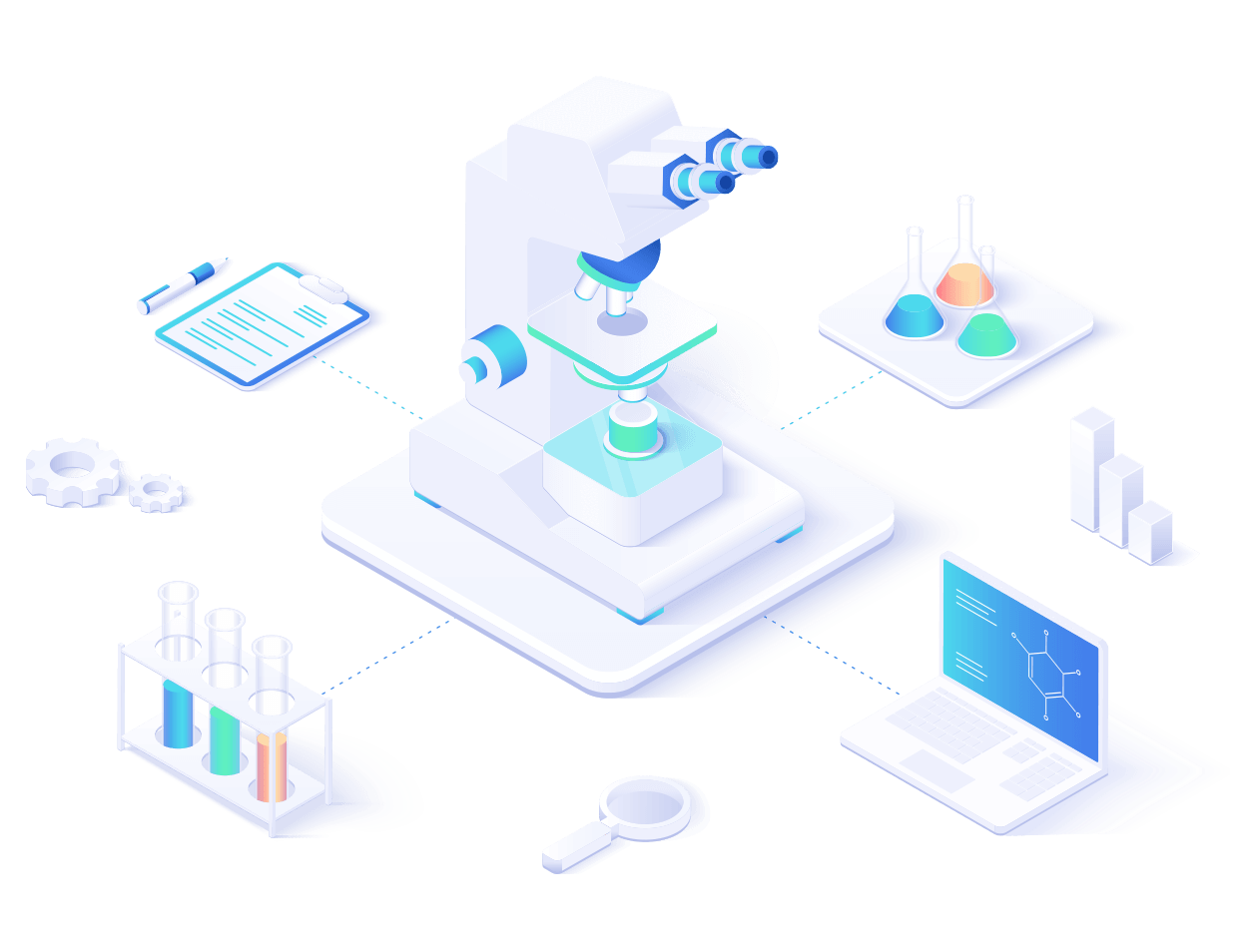
2016
Patent 2 Granted (CNM – the Comprehension Normalization Method for Semantic Language). The patent is a computer process designed to help cross-disciplinary analysis by allowing the same, initial piece of literature to be reconnected from the perspective of many disciplines.
Like people, data sets have unique languages and this method induces them to effectively rephrase the content of one from the unique perspective in language from/of the other.
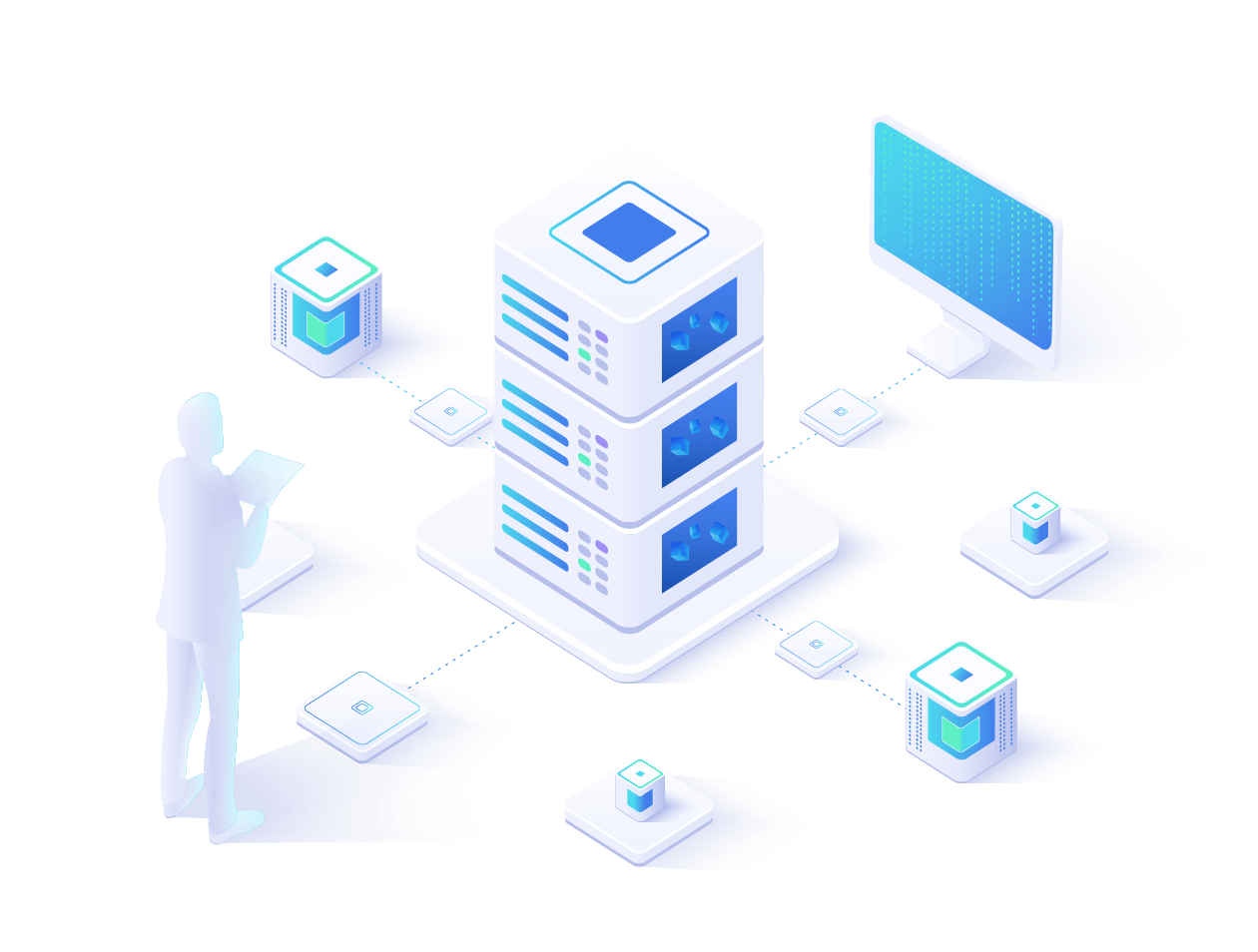
2016
CNM successful validation on genomic data studying obesity.

2016
2nd CNM successful validation, with Dr. Sophia Frangou: using imaging data (fMRI) from schizophrenia research.
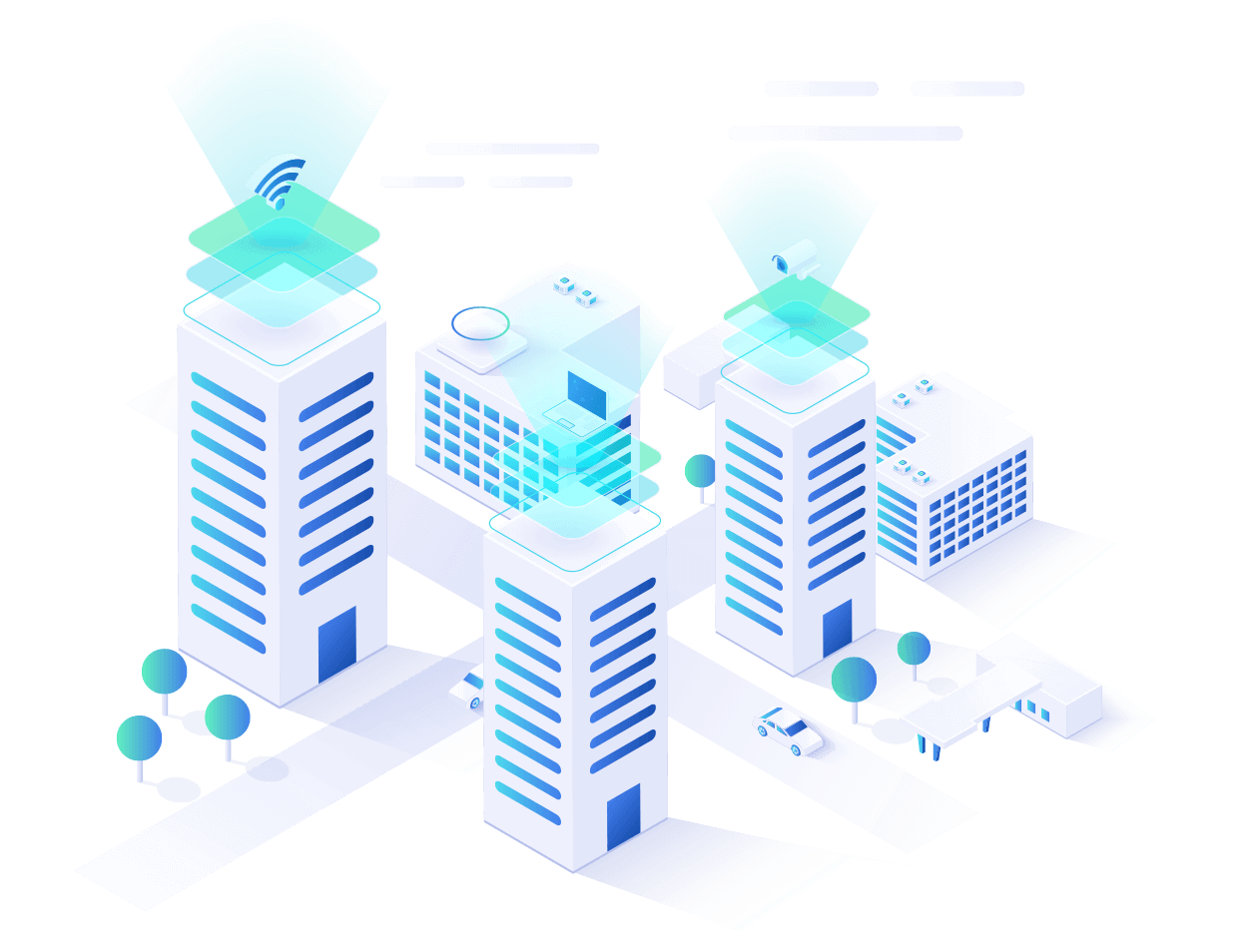
2018
Breakthrough Pilot with 19 coexpression networks data directed to from Eli Lilly and AMP-AD from patients that had had Late Onset Alzheimer’s Disorder.
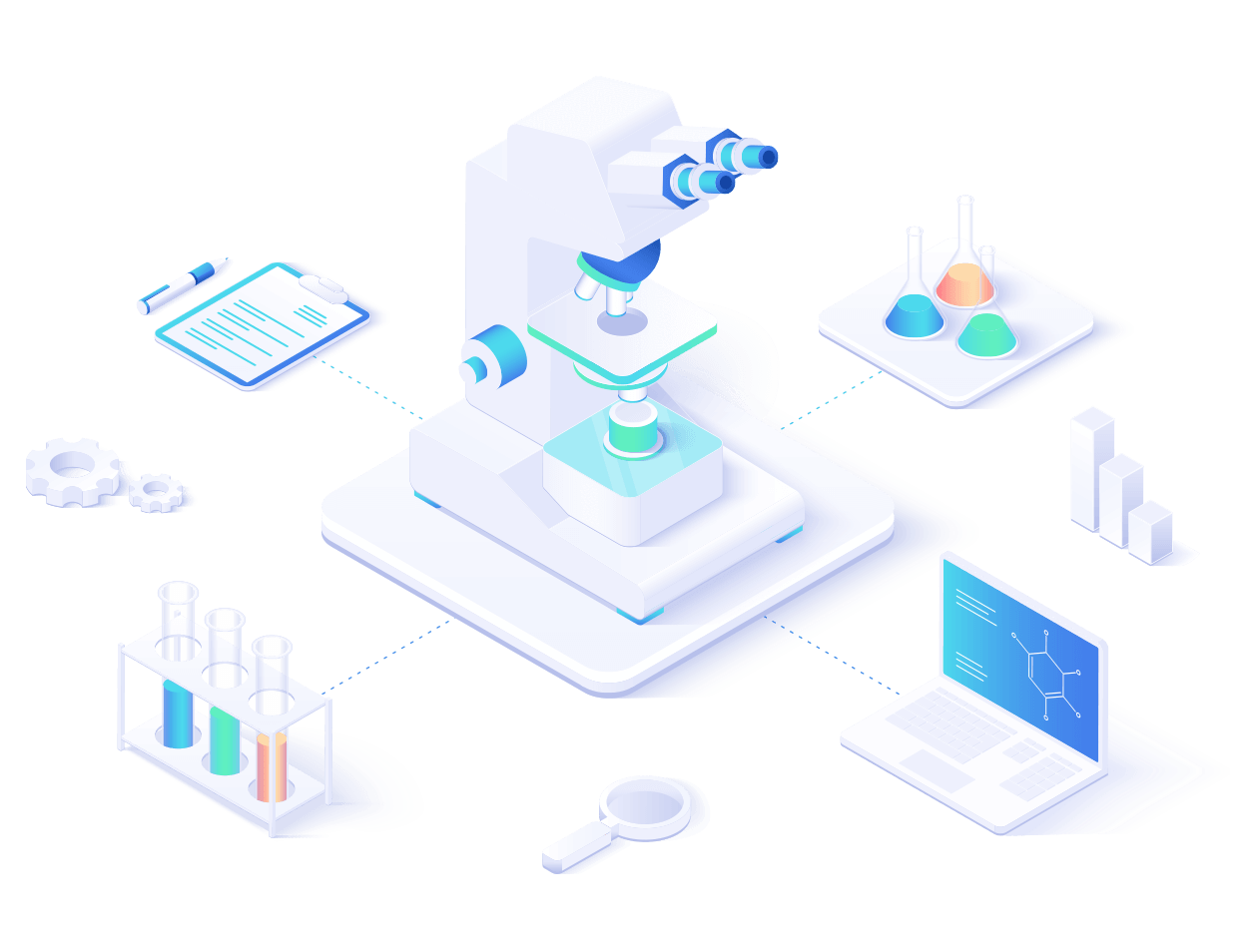
2019
Hyperfine moves into 600 3rd Ave NY, NY
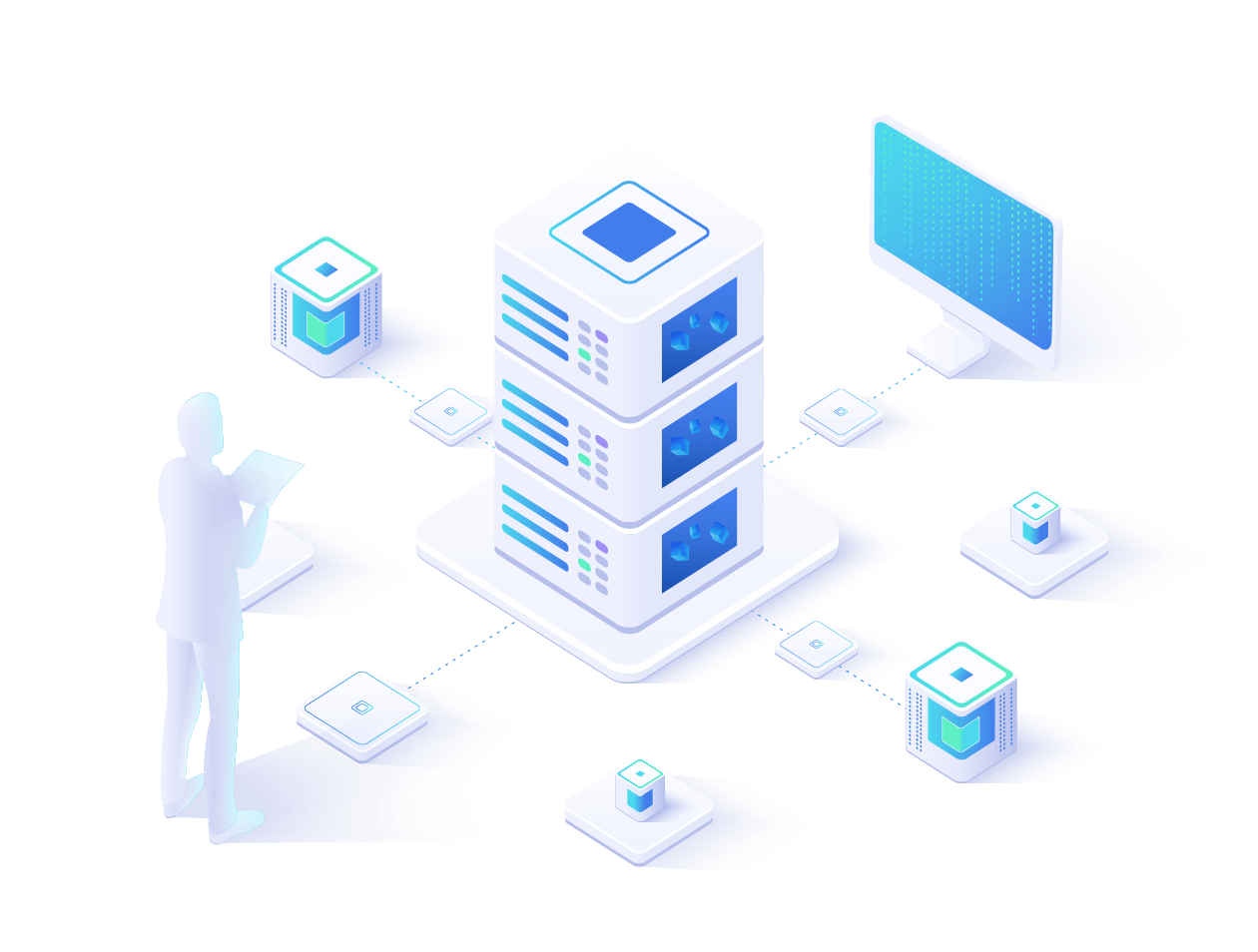
2020
Patent 3 Granted (CNM for Networks)
This is the CNM technology that can rapidly extract functionally related subnetworks at a speed so much faster than the integrative causal network constructing methods that with so many analyses a new level of information can be deduced. We consider this the potential scaling up of systems biology. Because extracting subnetworks from constructed causal networks is so computer power- and time intensive that extracting subnetworks from hundreds of scenarios is time prohibitive.
We have validated and are continually validating that the functionally related subnetworks identified by CNM are as accurate as the those relying on a fully constructed causal network. Identifying subnetworks over 1000 times faster makes it possible to evaluate subnetworks with each other to gain a higher order of information (like information about tissues from gene data).

2022
Begin using CNM with Language in Schizophrenia linguistic data
Management Team
CEO and Cofounder
Bridget Osetinsky has a unique background in both Physics, at University of Notre Dame, and Law at Temple Beasley School of Law. She filed for a patent for a laser that converges to a variable focal depth while in college, and is the inventor of Hyperfine’s current three technologies. She is also the recruiter of the rest of the management team of Hyperfine.
CTO and Cofounder
Dr. Abdul Aziz got his PhD in computer science from Southern Methodist University. He was formerly the Dean of Information Technology at a top technical school in Pakistan. He has a history of successfully leading software development projects, as the head of R&D and software development for Palmchip. Most recently he served as the CEO of an eCommerce business called Intellyct.
COO and Cofounder
Bobby Mannepalli is the COO and Cofounder. He studied history and communications at the University of Pennsylvania. He formerly worked in Investment banking for Distressed Mergers & Acquisitions with SSG Capital. He guides financial choices and how to communicate our technology.
.